Session 2C – Urban Analytics
Tuesday 30 March, 11:15 – 12:45 // Session Chair: Jinmo Rhee
051 – Hyperlinking Mechanisms in Commercial Complex: An Example of the Spatial Network in Taikoo Li Sanlitun, Beijing
Tuesday 30 March, 11:15, Session 2C
Shaoji Wu, School of Architecture, Tianjin University
Commercial complexes play an important role in contemporary cities, with elevators, escalators, and other paths on which people do not take natural movement in it. We consider them as spatial hyperlinking paths, which is originated from the web’s hyperlinking technology. This paper studies the path network system in Taikoo Li, Sanlitun, Beijing, in three steps. Firstly, The path system is transformed into a network model, and its spatial network distribution is characterized using betweenness centrality. Secondly, a deep learning approach is used to measure the people’s flow at the selected 102 observation points. Then a multiple linear regression(MLR) analysis is conducted using the flow data as dependent variable. And there are 7 independent variables in three types, including betweenness centrality C, H1 and H2 that related to spatial hyperlinks, and B1, F1, F2, and F3 that related to floors. Thirdly, analyzing the MLR model.There are two conclusions. First, using multiple independent variables is better than one variable to fit the people’s flow distribution using the regression model. Second, escalators have the effect of enhancing people’s flow, while elevators have the opposite effect.
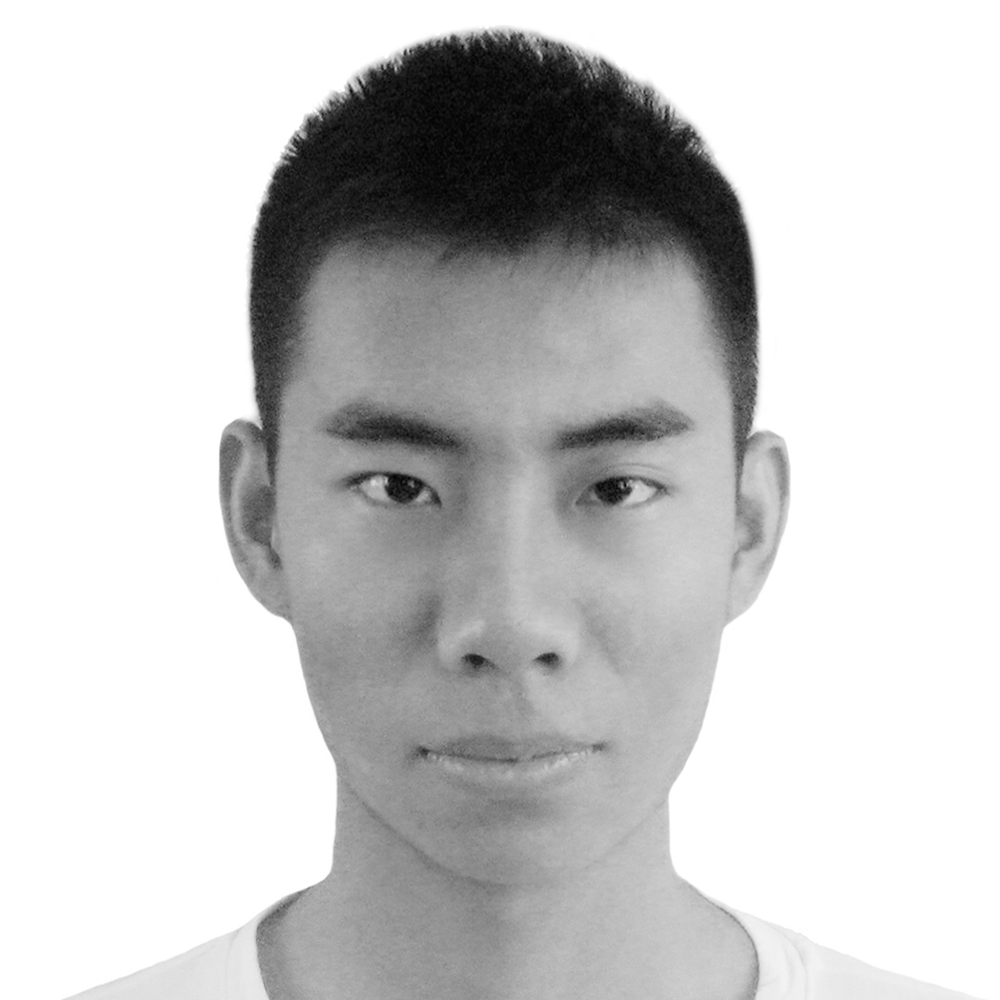
Wu Shaoji received the B.Arch. degree in School of Architecture from Tianjin University, Tianjin, China, in 2019. I am currently working toward the M.Sc. degree in Theory of Architecture Design with the School of Architecture, Tianjin University. My research interests include network science and environmental psychology.
135 – Archibase: A City-Scale Spatial Database for Architectural Research
Tuesday 30 March, 11:30, Session 2C
Yichen Mo, Southeast University
The explosion of geolocation data and data-based algorithms has the potential to analyze sophisticated urban areas and foster a more robust urban model. To better collect and organize the city data, this paper introduces a city-scale spatial database called ArchiBase, built upon Java and web APIs of open source databases. With hierarchical, layered, and regularly-updated spatial data defined by relation table, ArchiBase allows indexing and geometric searching of the entire city and supports applications and extensions for different cities. This research is from a graduate urban design course aiming to renew Prato, an industrial city in Italy. ArchiBase first creates the base version of Prato from multiple data sources, then illustrates the usability and expandability through three simple applications. The use of ArchiBase can better interpret future cities and demonstrate the unparalleled opportunities of collaboration and remote work for urban researchers and designers.
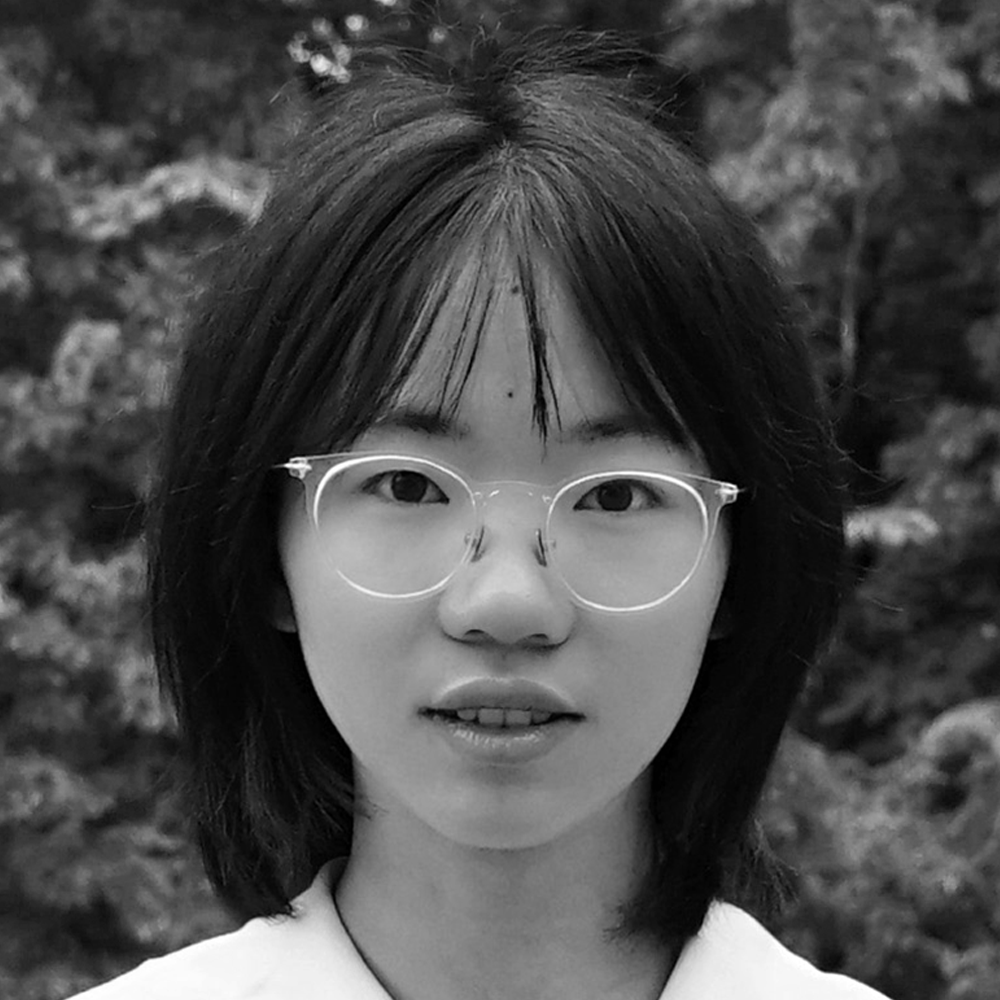
MO Yichen is currently a Ph.D. student at School of Architecture, Southeast University, advised by Prof. Biao Li. Her research is in architectural representation learning and geometry processing, designing new algorithms and data structures for architectural data.
081 – Sensitivity Analysis of Pedestrian Simulation on Train Station Platforms
Tuesday 30 March, 11:45, Session 2C
Danrui Li, Tongji University
Rong Huang, Tongji University
Yihao Wu, Tongji University
As the concerns for pedestrian safety in station design are growing, multi-agent simulation becomes more widely used nowadays.While the difference between inputs in regard to their impacts on simulation outputs needs further research, previous studies fail to provide a global analysis of it in complex environments with limited computation resources. Therefore, regression-based SRC and revised Morris Method are employed in a sensitivity analysis of train station platform simulations. Results show that preference for escalators and alighting rate are influential parameters to all three concerned outputs while the standard deviation of walking speed is negligible. Given that most simulation users have limited time and resources, this paper provides a list of parameters that deserve the time and effort to calibrate together with a factor fixing method that can be applied in similar scenarios. In this way, simulation users can lower the uncertainty of train station simulations more efficiently.

LI Danrui is a postgraduate in the College of Architecture and Urban Planning, Tongji University, with a Bachelor’s degree in Architecture from Chongqing University. His research interests range from multi-level pedestrian modeling to quantitative environmental behavior study. He is currently working on his master thesis on pedestrian simulations and evaluations for train station design. The thesis objective is to derive design principles for train stations that deeply integrate with urban activities and advanced technologies.
HUANG Rong is a master student in the College of Architecture and Urban Planning, Tongji University, and a member of Joint International Research Laboratory of Eco-urban Design, Tongji University. Her research focuses on data-informed urban design. Rong’s work including innovations in Urban Morphology of planning, digital cartography and integrating multi-sourced new urban data with machine learning. She is now committed to combining new data with traditional design needs, and introducing quantitative analysis into urban design and analytical research. Rong co-authored multiple papers on Urban Foresty & Urban Greening, Time+Architecture, Landscape Architecture and Chinese Landscape Architecture.
Wu Yihao is an academic graduate student in College of Architecture and Urban Planning, Tongji University. The research focuses on the quantitative analysis of architecture and urban space, spanning multiple scale levels of built environment. He has published nearly ten papers in national core journals, devoted to promoting the rationalization and scientization of architectural design methodology. In the field of architectural practice, the leading and participating design projects cover interior design, architectural design and urban design, with rich engineering experience.
310 – Global Urban Cityscape – Unsupervised Clustering Exploration of Human Activity and Mobility Infrastructure
Tuesday 30 March, 12:00, Session 2C
Tania Papasotiriou, The University of Newcastle, Australia
Stephan Chalup, The University of Newcastle, Australia
It is widely accepted that cities cultivate innovation and are the engines of productivity. The identification of strengths and weaknesses will enchant social mobility providing equal opportunities for all. The study at hand investigates the relationship between social mobility and transportation planning in 1,860 central urban areas across the globe. Datamining processes combining open-sourced, automated, and crowdsourced information from four major pillars of social mobility (demographics, human activity, transport infrastructure, and environmental quality) are used to describe each location. Next, unsupervised clustering algorithms are used to analyse the extracted information, in order to identify similar characteristics and patterns among urban areas. The process, which comprises an objective framework for the analysis of urban environments, resulted in four major types of central areas, that represent similar patterns of human activity and transport infrastructure.
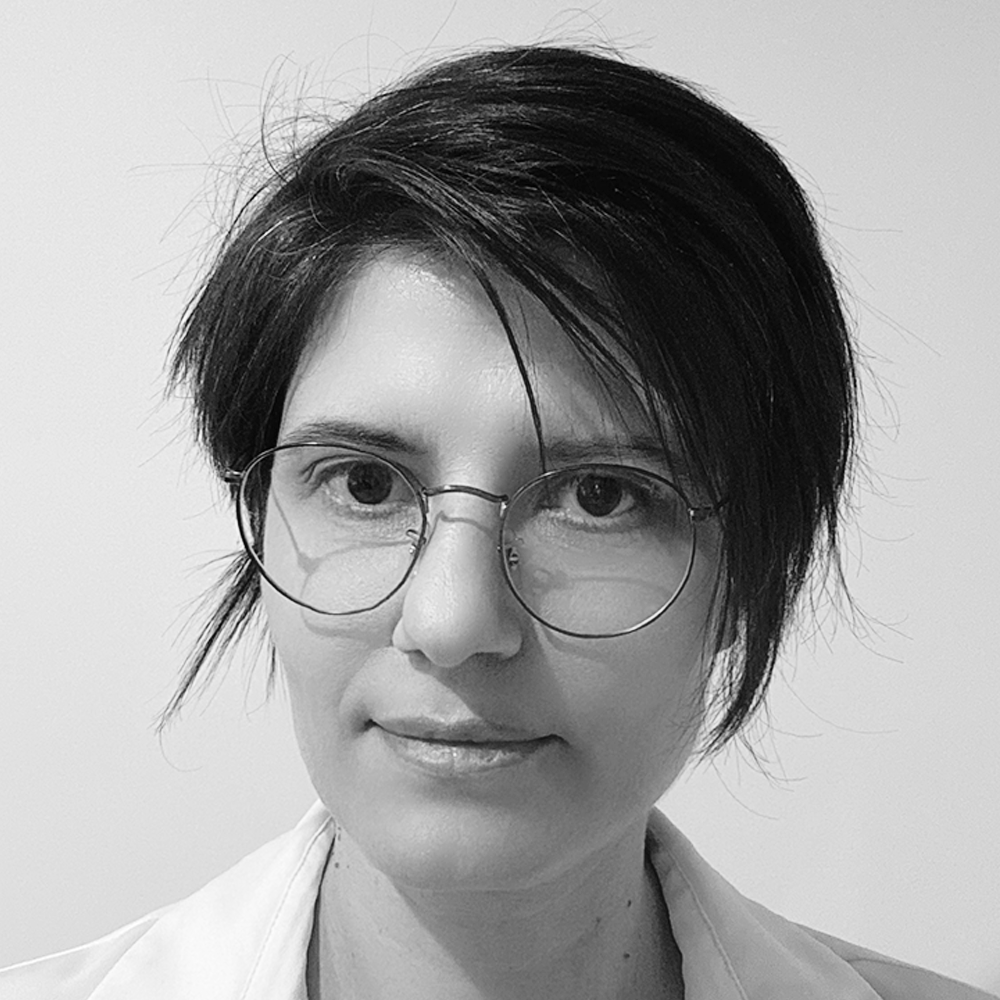
Tania Papasotiriou is a PhD candidate at the University of Newcastle in Australia where she holds an Industry Educator position. Her research investigates the implementation of Autonomous vehicles and their impact on build environments. Tania was practising Architecture in Greece for more than 10 years before she moved to Australia and she has showcased her work in n a number of exhibitions in Europe and Australia.
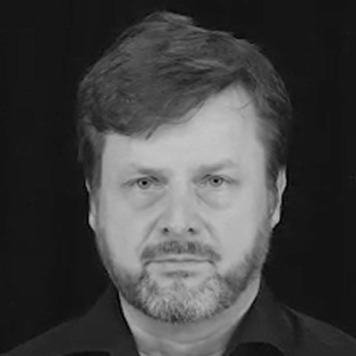
I received my PhD in 2002 from Queensland University of Technology where I studied at the Machine Learning Research Centre. Currently, I am an Associate Professor in Computing and IT at the University of Newcastle in Australia where I lead the Interdisciplinary Machine Learning Research Group and the Newcastle Robotics Lab. My research interests include artificial neural networks, topological data analysis, and various aspects and applications of artificial intelligence and machine learning. I have extensive experience from various projects in academia and with industry, where my largest collaborations were with architecture and with transport safety in the rail industry.
354 – Comprehensive Analysis of the Vitality of Urban Central Activities Zone Based on Multi-Source Data
Tuesday 30 March, 12:15, Session 2C
CHENYU HUANG, School of Architecture and Art, North China University of Technology
PIXIN GONG, School of Architecture and Art, North China University of Technology
RUI DING, School of Architecture and Art, North China University of Technology
SHUYU QU, School of Architecture and Art, North China University of Technology
XIN YANG, School of Architecture and Art, North China University of Technology
With the use of the concept Central Activities Zone in the Shanghai City Master Plan (2017-2035) to replace the traditional concept of Central Business District, core areas such as Shanghai Lujiazui will be given more connotations in the future construction and development. In the context of today’s continuous urbanization and high-speed capital flow, how to identify the development status and vitality characteristics is a prerequisite for creating a high-quality Central Activities Zone. Taking Shanghai Lujiazui sub-district etc. as an example, the vitality value of weekday and weekend as well as 19 indexes including density of functional facilities and building morphology is quantified by obtaining multi-source big data. Meanwhile, the correlation between various indexes and the vitality characteristics of the Central Activities Zone are tried to summarize in this paper. Finally, a neural network regression model is built to bridge the design scheme and vitality values to realize the prediction of the vitality of the Central Activities Zone. The data analysis method proposed in this paper is versatile and efficient, and can be well integrated into the urban big data platform and the City Information Modeling, and provides reliable reference suggestions for the real-time evaluation of future urban construction.
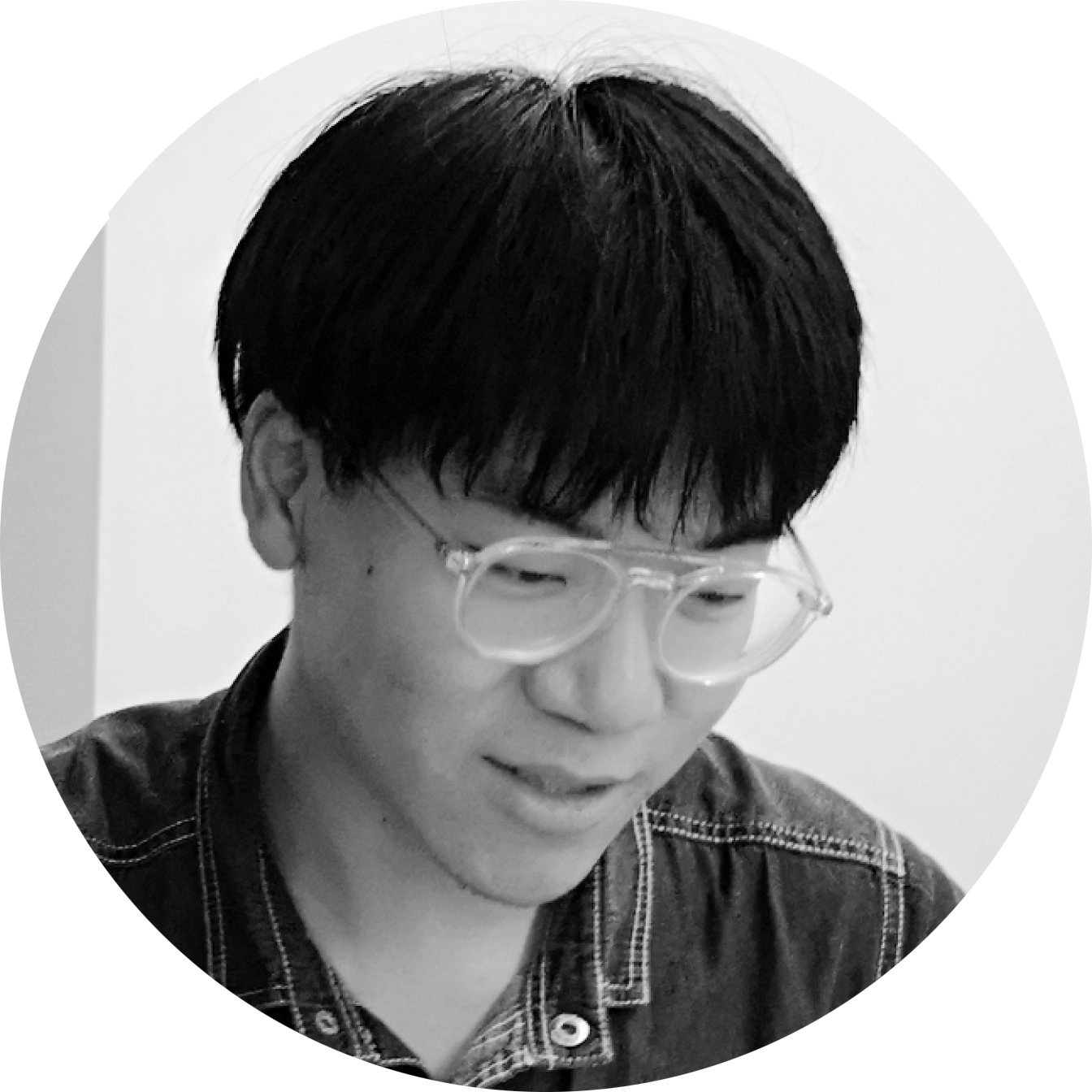
CHENYU HUANG, Master of Architecture from North China University of technology. He graduated from Xi’an University of Architecture and Technology. The traditional engineering education provided him a good foundation in mathematics and computer science. After graduation, he worked as an intern in algorithm development and robot fabrication in Tongji University and Fab-Union. He often participated in Shanghai DigitalFUTURES workshop held by Tongji University. And his interests include the application of AI and big data in quantitative analysis of urban morphology, performance-oriented generative design, etc. His works have been published in Building Science, Architecture Technique and the proceedings of CAADRIA.